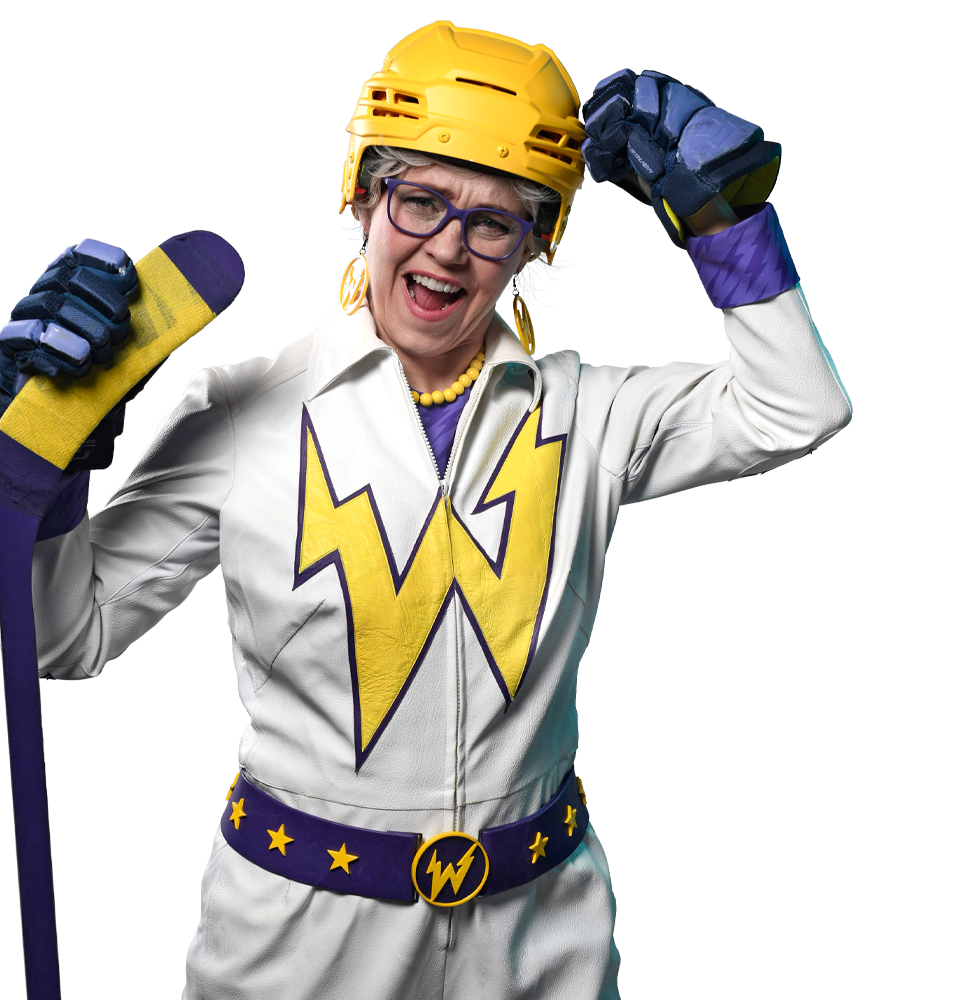
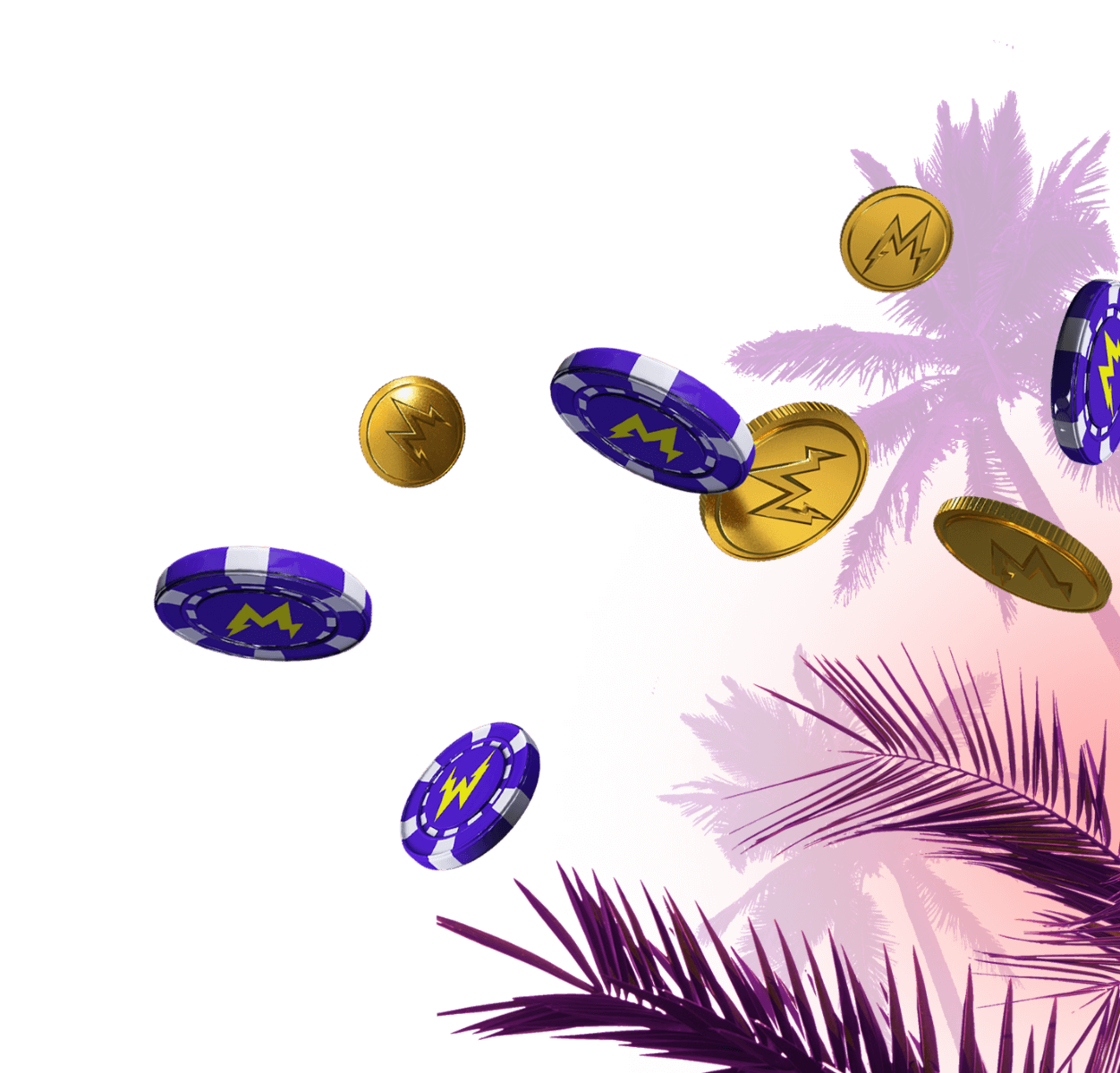
You can drag me around
Swipe up to continue playing
Some football fans hate it, while others swear by it. And no, we’re not talking about VAR. Actually, we’re about to discuss expected goals or xG. Is it a genius philosophy or a flawed statistic? Please continue reading to find out what xG means, how it’s calculated and why it divides opinion amongst the football community.
A relatively new concept in the sporting landscape, xG is a measure of the difficulty of a goalscoring opportunity in Football. In other words, expected goals measure the quality of goalscoring chances and the likelihood of them being scored. xG uses historical data from a large backlog of similar shots with common characteristics to estimate the likelihood of a goal on a scale between 0 and 1.
So, the easier the goalscoring chance, the higher the xG. The harder the chance, the lower the xG. For example, a Lionel Messi shot on goal from ten yards away will more likely have a higher xG than a shot from 30 yards. Whereas his chance from ten yards may have an xG of 0.55, i.e., a 55% probability, his xG from 30 yards could have an xG of 0.05 or a 5% probability.
So let’s say during the course of that game Messi only had these two shots, but he scored one goal. Therefore his goals to expected goals ratio would be 1 to 0.6.
But xG is more complex than just measuring a player’s distance from the goal. That’s because there are many factors that go into determining a player or team’s xG.
As every xG model has its own set of rules, it can sometimes be difficult to get a true gauge on xG. Fortunately, most xG models have certain tangibles in common. In addition to the distance to goal, these factors include:
Further to this mix of circumstances, xG also looks at tons of historical data from past games.
So, for the previously mentioned two Messi attempts on goal, a typical expected goals model will usually look at his and other players’ historical data in similar situations.
In the football world, many people believe that expected goals are the most accurate metric in predicting team and player performance. xG datasets allow everyone from professional bettors to sportsbook odds compilers to look beyond the outcomes of matches and get a better insight into the implied quality of both teams and players moving forward.
So, compared to statistics like ball possession and shots on target, xG gives a clearer picture of what a team or player was able to achieve (or not).
Looking back at the last World Cup, one of the most memorable results was Argentina losing 1-2 to Saudi Arabia.
While the Argentines had 70% possession, it doesn’t reflect the real nature of the game. Digging into the xG for both teams. 2.24 (Argentina) to 0.14 (Saudi Arabia), you can see that the Saudis overachieved in front of goal.
In addition to converting two “difficult” chances, Saudi Arabia also outsmarted Argentina with a daring high line-offside trap and constant pressing.
With so much data to digest, proponents of xG are always looking for better ways to present its information. That’s why many statistical companies have come up with clever illustrations like xG maps. An expected goals map can help everyone, from fans to head coaches, analyse team and player performance over a given period of time.
For example, an xG map can help put Erling Haaland’s 2022/2023 record-breaking Premier League debut season into perspective when he had a staggering goals to expected goals ratio of 36 to 28.7.
An xG map can visualise these numbers by further illustrating:
An xG map can also measure his expected assists (xA), i.e., measuring the likelihood that his completed pass will become a goal assist. Even better, metrics like xA and xG could create new ones like expected goal involvements and beyond.
Yes and no. At the end of the day, xG, just like possession and chances created, is one metric out of many. Just as Wildz Sports has access to xG figures and tables, so do sports bettors.
By comparing the xG values of shots taken by teams and players with their prop odds, it’s possible to identify value bets. For example, if a team has a high xG like Newcastle United in 2023/2024 but has long odds to win a match, there could be some value in that wager.
But relying on xG alone would be a mistake on both sides of a wager. That’s because sportsbooks factor in a whole host of other factors when determining the odds for certain.
That’s why it’s important for sports bettors to always undertake their own research to study form, injury news, xG, etc, before placing a bet.
Another reason not to take xG as gospel is because it’s a very subjective metric.
We’ve looked at the efficiency of xG now for the downsides. As mentioned before, xG, by nature, is very subjective. That’s because, for every Erling Haaland, there’s a Timo Werner. Sorry, Timo. Using raw data is one thing, but how you interpret it is another.
Let’s imagine the sporting director of Arsenal is looking for a new striker and is considering buying either Haaland or Werner. To do this, he compares their production in the Premier League given that Haaland has currently played 66 games in the competition to Werner’s 69 for Chelsea and Tottenham Hotspur.
While Haaland has smashed records at Manchester City with his ratio of 63 to 64.41, Werner has disappointed with a mere 12 goals to 23.81 xG. It’s clear that Haalaad has outperformed his xG, but Werner has underachieved his.
This tells us that “at Arsenal”, with their xG for every shot being the same, Haaland will probably have a higher conversion of xG into actual goals versus Werner. But in reality, Haaland will usually always have a higher xG because he will also get into more scoring opportunities. But xG isn’t that night and day.
What xG can’t take into account are factors like the impact of coaching, tactics, teammates, variance, bad luck, pressure and potential injuries. These factors might not seem like much, but over a 60-something-game period, they could begin to add up and start making a difference.
The jury seems to be unanimous, and xG is here and here to stay. But what does the future look like for football analytics?
As we mentioned before, does combining expected goals and expected assists give rise to expected goal involvements? What about expected interceptions, dribbles, tackles, fouls, saves and beyond.
While it may seem over the top, these new variants will be key to improving player monitoring and development, artificial intelligence and machine learning, which in turn will improve the xG model further.